Evaluating Statistical Significance
As Maury Povich says, "The results...ARE IN!" Now what?

Did You Know? 64% of you opened and read yesterday’s formula-dense email about calculating sample sizes! I know you didn’t know that—because how could you?—but I am seriously impressed! 👏
Let’s ride that wave of momentum 🌊 and finish off yesterday’s two-part topic that you probably didn’t know was two parts until right now.
Yesterday: Calculating minimum sample size for A/B split tests
Today: Evaluating your results and testing for statistical significance! 🥳
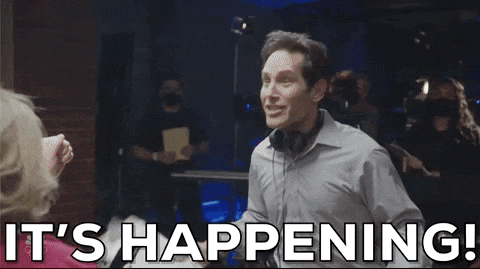
Gif by snl on Giphy
To recap, imagine you’re an email marketer testing which subject line generates a better email open rate. Here’s the situation:
You’re sending to a list of 2,000 subscribers.
1,000 subscribers will get Variant A and 1,000 subscribers will get Variant B.
Historically, your emails have a 20% open rate.
Yesterday, we used some really-not-that-difficult math to determine the minimum required sample size to get statistical reliability with our A/B test of email subject lines.
That math told us we’d need to send each variant to at least 246 subscribers. We’re sending each variant to 1,000 subscribers, so we’re golden.
Empowered by our statistical prowess, we finalized our email campaigns and sent them off.
It takes about three days to get a complete picture of an email marketing campaign’s performance.
I debated saving this topic for Thursday to make it all feel more realistic, but then I realized that’s ridiculous. Why wait when we have movie email magic?
Someone call Maury Povich, because the results are in.

Each variant was sent to 1,000 subscribers.
Variant A’s subject line was written in your company’s typical style.
Variant B’s subject line added personalization.
Did it work? Here are the stats:
Metric | Variant A | Variant B |
---|---|---|
Emails Sent | 1,000 | 1,000 |
Emails Opened | 200 | 220 |
Email Open Rate | 20% | 22% |
Note: We’re only looking at open rate to keep this example simple, but remember the difference between vanity vs action metrics. While email open rates generally correlate with increased clickthroughs and downstream revenue, higher open rates don’t guarantee better financial performance. It’s important to understand your company’s business objectives when choosing how to measure the success of any marketing campaign.
Variant B had a 10% higher open rate (22% vs 20%). That means the personalized subject line worked, right?
To say for sure, we need to determine the statistical significance of the results.
To do that, we need to do...more math!

Whoa, whoa, whoa…
“Bryan, you lying sack of 💩. I thought we already determined our sample size was large enough. Why do we need to test the results at all?”
There are two key steps to perform when analyzing the results of an A/B split test:
Step 1: Determining the minimum sample size for statistical reliability
Step 2: Calculating the statistical significance of the results
Yesterday, we performed Step 1: Determining the minimum sample size for statistical reliability. This step ensures we have enough data to be able to confidently calculate whether our results are statistically significant. That’s important, because if our sample size is too small, we run a higher risk of encountering two types of error with super creative names:
Type I Error: Falsely claiming significance (false positive)
Type II Error: Failing to detect a real effect (false negative)
This visual about the difference between Type I and Type II error has always been one of my favorite data memes:
Today, we’re performing Step 2: Calculating the statistical significance of the results. Because of yesterday’s work, we’re confident that our sample size is both (a) large enough and (b) random enough to be able to determine whether our Variant B personalized subject line has a real, significant effect on our email open rate.
Determining statistical significance
There are a couple ways to do this—either a z-test or a t-test. We’ll use a z-test where “z” refers to standard deviations, just like yesterday. These are the steps:
State the null and alternative hypotheses
Calculate the pooled proportion
Calculate the standard error (SE)
Compute the z-score
Evaluate the z-score
Don’t worry about all the mathy jargon. It sounds more complex than it is.
1. State the null and alternative hypotheses
At its most basic, A/B testing is a form of hypothesis testing. In this example, our hypothesis is that Variant B’s personalized subject line will increase email open rates. There are only two possible outcomes: false or true. Those are our two hypotheses:
Null hypothesis (H0): There is no difference in open rates between Variant A and Variant B (pA=pB).
Alternative hypothesis (Ha): There is a difference in open rates between Variant A and Variant B (pA≠pB).
pA is the open rate for Variant A (20%) and pB is the open rate for Variant B (22%).
2. Calculate the pooled proportion
The pooled proportion is a fancy term for the weighted average open rate between Variant A and Variant B as calculated by the following formula:

Keeping with tradition, it’s really not as complex as it seems:
p: The pooled proportion (weighted average) of A and B’s open rates
pA: Variant A’s open rate (20%)
nA: Variant A’s sample size (1,000 sends)
pB: Variant B’s open rate (22%)
nB: Variant B’s sample size (1,000 sends)
Because we’re using an easy example, the pooled proportion is 21%, which we’ll write as 0.21.
3. Calculate the standard error (SE)
The standard error is a measure of the variability of our data around its mean.
You may be asking yourself, “How can we calculate the variability if we only have the average email open rates of Variants A and B (20% and 22%, respectively)?”
(Or you may not be asking yourself that. That’s cool, too.)
The reason we can calculate the standard error is because z-tests assume we’re dealing with a normal distribution of data, which means our email open rates distribute themselves on a bell curve like this…

…where the average is in the middle and both the left and right sides of the curve are mirror images of each other.
That’s a pretty fair assumption about email open rates, but you can determine this for yourself using your own historical data.
If you find your email open rates are not normally distributed like the bell curve above, then you should use a t-test to determine statistical significance and not a z-test like we’re using here.
Here’s the formula for our standard error calculation:

And here are the definitions of each variable:
SE: The standard error (what we’re calculating)
p: The pooled proportion (weighted average) of A and B’s open rates
nA: Variant A’s sample size (1,000 sends)
nB: Variant B’s sample size (1,000 sends)
Let’s plug our values into the formula…

And then do some easy math…
Voila! Our standard error is 0.0182.
4. Compute the z-score
A z-score is a measure of the number of standard deviations separating two values. To calculate the z-score for our data, we’ll use our open rates for Variants A and B to determine the magnitude of the absolute difference between those open rates, and then use the standard error we just calculated to determine the magnitude of that absolute difference as it relates to a normal distribution of email open rates.
Translation: We’ll see how far apart Variant A and B’s open rates are and then determine whether that’s a big gap or a small gap.
The formula:

And the definitions:
z: The z-score (standard deviations separating Variants A and B)
pA: Variant A’s open rate (20%)
pB: Variant B’s open rate (22%)
SE: The standard error (what we just calculated, 0.0182)
Plug in the values:

More math:
Ta-da! The z-score for our A/B test is -1.10.
Our z-score being negative doesn’t really matter. The magnitude of the difference between the open rates of Variant A and Variant B is 1.10 standard deviations. The negative sign tells us the directionality—that Variant B had a higher open rate than Variant A.
5. Evaluate the z-score
We have our z-score for this A/B test: -1.10.
Now what?
To determine statistical significance at a 95% confidence level, we look for a z-score that lies outside the range of -1.96 to 1.96.
Why 1.96? Because in a normal distribution, 95% of the data falls within ±1.96 standard deviations of the mean.
Our z-score of -1.10 does NOT lie outside the range of -1.96 to 1.96, which means the difference in open rates between Variant A (20%) and Variant B (22%) is NOT considered statistically significant.
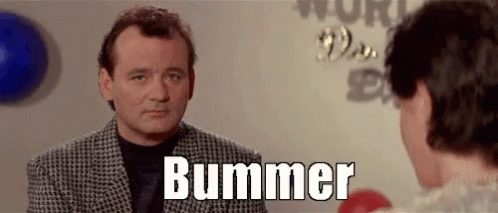
Giphy
That means we can’t say with at least 95% confidence that Variant B’s increased open rate is due to the personalized subject line.
But remember: That doesn’t mean Variant B’s increased open rate isn’t due to the personalized subject line. It just means we can’t say that with at least 95% confidence.
For the sake of completeness, at what open rate could we have said with at least 95% confidence that Variant B’s improved performance is due to the personalized subject line?
It just so happens I made a table for several Variant B open rates.
Open Rate | Weighted Avg (p) | SE | Z-Score |
---|---|---|---|
22.0% | 21.00% | 0.0182 | -1.10 |
22.5% | 21.25% | 0.0183 | -1.37 |
23.0% | 21.50% | 0.0184 | -1.63 |
23.5% | 21.85% | 0.0184 | -1.90 |
23.6% | 21.80% | 0.0185 | -1.95 |
23.7% | 21.95% | 0.0185 | -2.00 |
If Variant B’s open rate had been at least 23.7%, we could have said with 95% confidence that the increased open rate was due to the personalized subject line.
For now…

Everyone say, “Hi!” to Henry L 👋
Question: What’s the most bizarre keyword you’ve ever optimized for in SEO?
Henry L’s Answer: “In my first marketing job I worked as a copywriter for an agency and was assigned to a client trying to optimize for lots of longtail keywords with short FAQ blog posts. I had to find threads in forums where people were asking related questions and then write 200+ word articles answering those questions. My client was in the finance niche so I wrote a lot of articles about banks and credit cards. One question I had to write a serious article answering was ‘Can I leave my estate to my snake?’ Not just pet, but snake. I didn’t stay at that job for very long!”
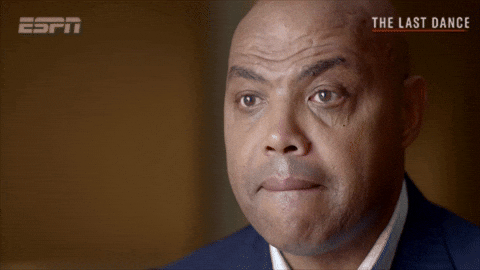
Gif by espn on Giphy
ChatGPT-Generated Joke of the Day 🤣
Why did the coffee file a police report?
It got mugged.
Suggest a topic for a future edition 🤔
Got an idea for a topic I can cover? Or maybe you’re struggling with a specific marketing-related problem that you’d like me to address?
Just reply to this email and describe the topic.
There's no guarantee I'll use your suggestion, but I read and reply to everyone, so have at it!