Super-Simple Sample Size Calculation
You know more data > less data, but when do you have ENOUGH data? Sample size to the rescue!

Did You Know? Just 19% of marketers rate themselves as having a high degree of data literacy. (Source)
More data > less data.
Data quality issues aside, I don’t think anyone would disagree with that.
But when does “more data” become “enough data”?
Imagine you’re an email marketer testing which subject line generates a better email open rate. Here’s the situation:
You’re sending to a list of 2,000 subscribers.
1,000 subscribers will get Variant A and 1,000 subscribers will get Variant B.
Historically, your emails have a 20% open rate.
Is your email list big enough to draw any conclusions from a single A/B test of two different subject lines?
We’ll answer that today, as the subject of this edition of Data-Driven Marketing is sample size.
This is the formula we’ll use:

Sample size formula
This is not an April Fool’s Day joke. We’re actually going to do this.
But let’s make it simple.
How to calculate required sample size
That scary-looking formula really isn’t so scary. Here’s what each variable means:

Sample size formula
n: Required minimum sample size (what we’re solving for)
Z: Z-score corresponding the confidence interval we set, usually 95% (more on this below)
p: Population variability, basically meaning our baseline performance (so 20% or 0.2 in this example)
E: Margin of error, which is 1 minus our confidence interval (95%) so 5% margin of error or 0.05.
Click here to jump past the upcoming explanation of Z-scores and confidence intervals.
Quick detour: Z-scores and confidence intervals
This is the toughest part of understanding sample size. Confidence intervals are our desired degree of certainty that any differences between the email open rates of Variant A and Variant B are due to the subject lines themselves and not random chance. A 95% confidence interval is pretty much the standard and assumes that there’s just a 5% chance that any results we see are due to random chance.
Z-score is a conversion of that 95% confidence interval to a different scale: standard deviations. In a normal distribution of data (like the bell curve in the image below, where both sides of the curve to the left and right of the average look the same), you’ll always have 68.2% of your data points within 1 standard deviation and 95.4% within 2 standard deviations. We want a 95% confidence interval (not quite 95.4%) so our Z-score is 1.96, which is just below 2.
If you want more certainty, you increase your confidence interval, perhaps to 99%, but look at the equation above to see the impact of this change: Z2 in the numerator (top) gets larger while E2 in the denominator (bottom) gets smaller. This drives n (your required sample size) up, essentially exponentially, meaning you need a much larger sample size for each extra degree of confidence.
Let’s do the (really not that difficult) math
Let’s plug our values into the equation…

And then do some math…
Our required minimum sample size is 245.86. 🎉
Going back to our original scenario, we’re sending to 2,000 subscribers split evenly between Variant A and Variant B, so 1,000 subscribers receiving each. 1,000 is more than 245.86, so we’re in the clear!
Let’s add a wrinkle…
You know segmentation improves ROI, so instead of sending this A/B email campaign to your entire list of 2,000 subscribers, you want to send it to just the people who already have purchased your product. That’s 20% of your list, so 400 total subscribers (200 receiving each variant).
Well, 200 × 2 = 400 is less than the minimum sample size we just calculated (246 × 2 = 492). What does that mean for our A/B test?
Let’s perform…
Finite population correction
According to our previous calculations, we need to send each variant to at least 245.86 246 subscribers. That’s a minimum of 492 subscribers between both variants. Unfortunately, our target segment has just 400 subscribers, so we’re short.
Well, not exactly.
We did have a small problem with our initial sample size calculation that wasn’t material until now: Our minimum sample size (492) makes up an extremely large portion of our total subscribers (2,000). 24.6% of our total, to be exact.
When your minimum sample size is such a large percentage, you introduce more bias into your results. That’s because as you assign each subscriber to a variant, you remove them from the pool of all remaining subscribers, which changes the composition of your pool of remaining subscribers.
If you have a large pool of remaining subscribers, that change isn’t very noticeable. If you have a small pool of remaining subscribers, that change becomes more noticeable.
Think of it like picking teams for dodgeball in gym class. Each team needs to pick 10 players, and there are 25 kids in the entire gym class. As each kid is assigned to a team, the composition of the remaining players changes drastically until, by the end of the team selection process, there are just a few kids left (and a lot of them are Winstons).

If each team needs to pick 10 players and there are 200 kids in the entire gym class, the composition of the pool of remaining players doesn’t change as much each time a kid is selected. By the time each team of 10 is selected, there are still 180 kids left. Chances are there’s more than a few Timmys left unselected.
As a general rule, if your required minimum sample size is more than 5% of your total population (subscribers, in this example), then you want to make a quick finite population correction (FPC) to adjust the sample size downward. In our example, our sample size was 24.6% of our total population.
Note that this calculation isn’t required if you’re already above the minimum required sample size we calculated earlier, such as sending A/B emails to our entire list of 2,000 subscribers when the math says we need just 492 subscribers. This FPC calculation only decreases the minimum sample size required to reduce bias, so we’re A-OK if we’re already sending to more than that original 492.
However, in this wrinkle I’ve introduced, now we’re sending to just 400 total subscribers. That’s less than 492, so let’s check to see how that 400 stacks up against our required minimum sample size when adjusting it downward to remove bias from that 24.6% of total from above.
Here’s the formula for FPC, which is pretty straightforward:

N: Total population size (2,000 subscribers in our example)
ntotal: Total sample size across both variants (246 × 2 = 492)
That leaves us with this:

Doing that math gives us FPC = 0.80. This is our finite population correction, so we multiply our original sample size values (246 × 2 = 492) by 0.80 to calculate our new minimums, which accounts for the total population size problem we discussed with our dodgeball example.
Factoring in FPC, our minimum sample size should be 246 × 0.80 = 197 × 2 = 394.
This means we need to send each variant in our A/B campaign to at least 197 subscribers (394 total). Our 400-subscriber segment is good enough!
Again, please note that we could have calculated this new, adjusted value even if we were sending to all 2,000 subscribers, but this adjusted value always will be less than the initial sample size calculation, so there wasn’t any need.
If you have any questions about sample size or need help with your sample size calculations, reply to this email and I’ll help you out!
Everyone say, “Hi!” to Jack B 👋
Question: If you could make any rule for one day and everyone had to follow it, what would it be?
Jack B’s Answer: “If I could make any rule for one day and everyone had to follow it: everyone leaves work on time no matter what.”
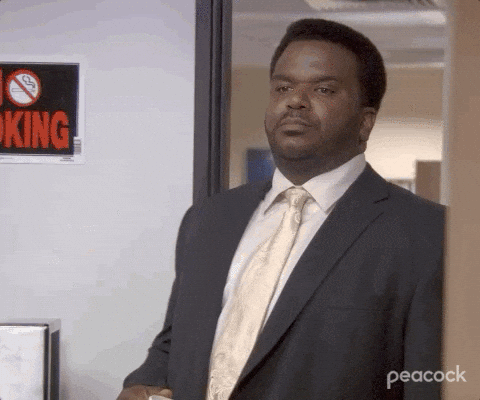
Gif by theoffice on Giphy
ChatGPT-Generated Joke of the Day 🤣
What's a scarecrow's favorite fruit?
Straw-berries!
Suggest a topic for a future edition 🤔
Got an idea for a topic I can cover? Or maybe you’re struggling with a specific marketing-related problem that you’d like me to address?
Just reply to this email and describe the topic.
There's no guarantee I'll use your suggestion, but I read and reply to everyone, so have at it!mple