AI Sentiment Analysis (and the Barbie Movie)
An easy-reading primer on a powerful AI tool. Plus Barbie.

Did You Know? As of 2022, 52% of large companies use sentiment analysis software vs. just 12% of small businesses. (Source)
For many marketers, AI triggers feelings of incompetence more than intelligence.
If that sounds like you, don’t beat yourself up. A 2024 survey found that a “lack of understanding of AI” is the number one challenge marketers face as they continue to integrate AI into their strategies. “Inadequate training or education” was right up there, too, with both responses earning more than 40% support.

But, given enough time and money, all challenges can be overcome. Unfortunately, time and money aren’t unlimited—is that a double negative? should I have said “are limited” instead?—so that same survey asked those same marketers which resources they felt were required for better AI integration. “More time to understand and implement AI” was the top choice at 62% with “more trained personnel” coming in second at 53%.

As you probably guessed based on the first 250 words of this email, today’s edition of Data-Driven Marketing focuses on AI.
And, as you probably guessed from the “Did You Know?” stat in the intro, we’re going to narrow that focus to sentiment analysis, otherwise known as opinion mining.
So grab your pick-axe and get ready to strut your stuff, because we’re going in.
What is sentiment analysis?
Sentiment analysis is a sub-category of natural language processing (NLP).
Other sub-categories of NLP include text classification (used in spam detection), machine translation (translating between languages), question answering (extracting answers to specific questions from a body of text), and more.
Wait, what is NLP?
My bad. NLP is a field of artificial intelligence that focuses on the interactions between computers and human language. It involves the development of algorithms and models that enable computers to understand, interpret, and generate human language in a way that’s both meaningful and useful. It’s what powers ChatGPT.
Got it. OK, back to sentiment analysis.
Sentiment analysis involves identifying and extracting subjective information from text data, such as the opinions, sentiments, and emotions expressed by real people. It’s frequently used to assist in the following tasks:
Social media listening for day-to-day monitoring or around specific events, such as product launches.
Analyzing survey responses for fast, large-scale reporting.
Processing employee feedback in large organizations.
Identifying unhappy customers so brands can intervene.
Monitoring trends to determine what’s hot (or not).
Competitor research for brands to see how they stack up.
Like most things AI, there’s a lot of nuance and variance to how sentiment analysis can be deployed.
For our purposes, just think of sentiment analysis as placing the general sentiment of a body of text somewhere along the negative-to-positive spectrum.
Sentiment analysis can be performed at four different levels:
Aspect-level
Phrase-level
Sentence-level
Document-level
Each level has broader scope than the last.
Heads up, we’ve got a figure from a scientific paper coming in hot.

Aspect-level sentiment analysis is the most abstract of the four. An "aspect” in this context is a discrete entity, thing, or category described in the text. For example, let’s say you’re using sentiment analysis to analyze restaurant reviews. Here’s one such review:
“The food at Monk’s Cafe is fairly priced and tastes pretty good, and the wait staff is super friendly, but there were these four people in a single booth shouting at each other during my entire meal.“
That one sentence includes multiple aspects, each of which has its own sentiment:
Aspect | Text | Sentiment |
---|---|---|
Food | “the food…tastes pretty good” | Neutral |
Price | “the food…is fairly priced” | Neutral |
Service | “the wait staff is super friendly” | Positive |
Atmosphere | “there were these four people in a single booth shouting at each other during my entire meal” | Negative |
The other three levels—phrase, sentence, and document—are a lot more self-explanatory.
How does sentiment analysis work?
I think it’s almost always helpful to understand how something works, so here’s a light-and-easy explanation of what’s happening:
1. Text Input // You’ve got some text you want to analyze.
2. Text Preprocessing // When you submit text to be analyzed by an AI sentiment analysis model, it looks like you just submit the text and the model spits out a response, right? Under the hood, the AI model needs to clean up the text, converting it to a standardized format (all lowercase), removing common words that add no value (such as “the” or “a”), and splitting the whole body of text into individual words to be analyzed (called “tokenization”).
3. Word Scoring // Once tokenized (split into individual words), each word is checked against a sentiment lexicon, which is like a big reference table that tells the model how positive or negative each word is. Words like “happy” or “excellent” might have positive scores while words like “sad” or “terrible” might have negative scores. The scores for each word in the sentiment lexicon are assigned during the model training process, which happens way before you actually use the model to analyze your text.
4. Aggregation // With each word scored individually, it’s time to aggregate those scores to determine the overall sentiment of the text at any of the four levels (aspect, phrase, sentence, or document). Aggregation can be done in a number of ways, such as evenly weighting the scores for each word or applying more complex models to weight some words more than others.
5. Thresholding // Once aggregation is complete, we have a numerical representation of your input text’s sentiment. Sometimes you want that number as the final result of your analysis, but sometimes you want to assign general categories, such as positive, neutral, or negative. To do that, you need to have thresholds for each category, allowing you to see where the numerical sentiment value from aggregation lands.
6. Output // Whether you want the numerical sentiment value, the category, or both, the final step is to output the result of the analysis for you to use.
If you’re dying for more detail, this is a good read.
If you didn’t understand anything I just said, don’t worry about it!
Now, let’s have some fun.
Using sentiment analysis to evaluate reviews of Barbie
This is something you can do at home (or at work, whatever, I’m not your mom). Here’s what you’ll need:
Text reviews of Barbie (or whatever movie you’d like, again, not your mom)
Here’s what you do:
Find a review to analyze. Preferably a longer one. Preferably from an unhinged viewer.
Copy the review text and paste it into the sentiment analysis tool.
Marvel at the results.
Here’s a review I’m going to analyze from Claire P, who was not a fan of the movie:

Claire rated the movie as just one star ⭐. Personally, I think Claire’s crazy. Barbie was hilarious. Margot Robbie and the rest of the cast were fantastic, but—and yes, I understand the sad irony of a man getting all the hype in a movie about feminism—Ryan Gosling stole the show.
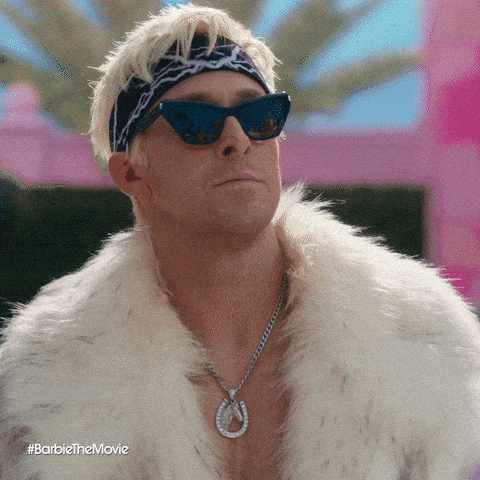
Gif by WBPictures on Giphy
Back to Claire’s terrible, objectively-incorrect “review.” Let’s see what the sentiment analysis tool says:
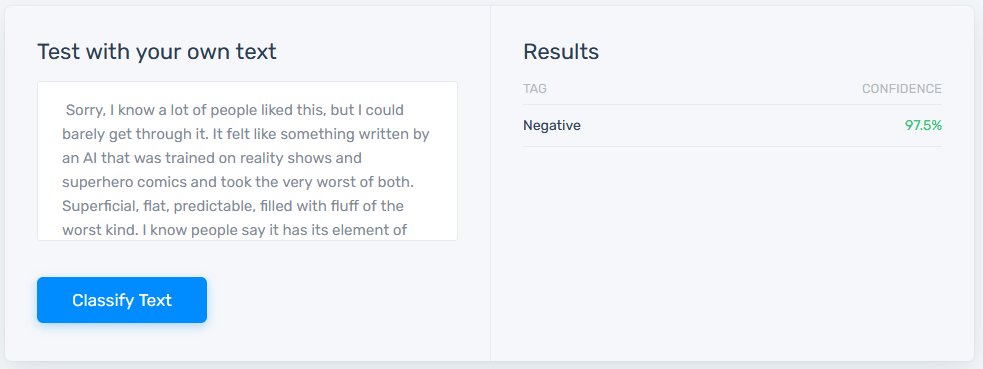
The tool classified the text as negative with 97.5% confidence.
C’mon, Claire.
With enough data, marketers could analyze the sentiment of every audience review of Barbie and do all sorts of cool things:
Analyze sentiment vs. demographic data to see how different age groups, genders, geographic regions, etc. responded to the movie.
Look at the sentiment for various aspects, such as individual actors or themes.
See how many of the 10,000+ analyses of individual reviews they can fit onto a single CVS receipt.
(It’s gotta be, like, at least 6,000, right?)
Look at everything we covered this week!
In case you forgot—or haven’t yet read, shame!—here’s what we discussed over the last week:
Monday: Above-the-fold optimization
Wednesday: The 4 marketing micro-moments
Thursday: Gen Z broke the marketing funnel
Friday: AI sentiment analysis (and the Barbie movie)
Got any questions about those subjects or want to suggest something for next week? Just reply to this email to let me know!
Everyone say, “Hi!” to Kevin P 👋
Question: If you could make any rule for one day and everyone had to follow it, what would it be?
Kevin P’s Answer: “Everyone must answer my cold calls.”
ChatGPT-Generated Joke of the Day 🤣
What's brown and sticky?
A stick!
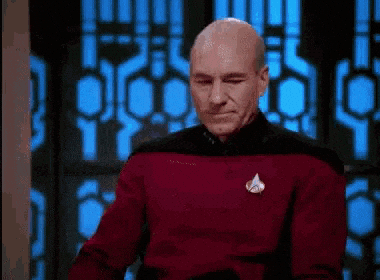
Giphy
Suggest a topic for a future edition 🤔
Got an idea for a topic I can cover? Or maybe you’re struggling with a specific marketing-related problem that you’d like me to address?
Just reply to this email and describe the topic.
There's no guarantee I'll use your suggestion, but I read and reply to everyone, so have at it!