CLV: The Most Valuable Marketing Metric (And One of the Hardest to Track)
Buckle up, folks. We're going in.

Did You Know? 76% of companies see Customer Lifetime Value (CLV) as an important concept for their organization, but just 42% can measure it accurately. (Source)
I’ve been meaning to cover CLV in one of these daily emails, and the “Did You Know?” stat from above, which you may recall from yesterday’s email about customer retention stats, finally convinced me to do it.
CLV is at the heart of almost all marketing campaigns, because it tells you how much a customer is worth to your business over their lifetime. That’s kinda important.
Here’s how CLV is calculated:
CLV = Customer Value x Customer Lifespan
And we can further breakdown Customer Value where…
Customer Value = Average Order Value x Purchase Frequency
Using some middle school algebra to replace Customer Value in the first formula, we can confidently say…
CLV = (Average Order Value x Purchase Frequency) x Customer Lifespan
Note to the “Well, technically…” crowd out there: I know the parentheses aren’t needed in the formula above. It’s for clarity. Get off my ass.
That formula looks simple enough, right? I mean, that’s only three variables! And you can probably rattle off some estimates for those values off the top of your head.
Not so fast…

CLV can be tricky to calculate or apply for several reasons:
Time periods, while not complex, can be tricky for some people to conceptualize. Average Order Value and Purchase Frequency are measured over a defined time period that makes sense for your business. Starbucks can measure those values using a shorter window than a furniture store.
Customer Lifespan probably is calculated over a different period, so you’ll need to do some conversions.
Calculating CLV requires comprehensive data collection across multiple channels and customer touchpoints, all of which need to be combined in a single place.
Even if companies can successfully aggregate their data, often there are questions about its accuracy or completeness.
At its foundation, CLV is about attribution, which is notoriously challenging for many marketers. 42% of companies still track attribution manually, which is nightmare fuel for me.
Even when companies can track attribution, there’s still the matter of segmenting customers. That’s important because…
You probably have several different types of customers, each of which has a different CLV. Larger companies with multiple product offerings targeting different audiences could have a ton of variance in the CLV of their various types of customers, but even smaller businesses with a single product can experience highly variable CLVs across different customer segments, such as acquisition source (organic search, podcast, TikTok, Instagram, etc).
CLV technically only measures the past while all marketing spend will be in the future, so you need to understand trends, seasonality, the competitor landscape, and more with an eye on the future.
Speaking of the past, CLV requires a long historical period (the “lifetime” part). Newer companies probably won’t have enough historical data to truly understand the lifetime value of their customers. Even if companies are more established, there’s a good chance their reporting systems aren’t, which means data becomes more sparse the further back you go.
And let’s say you’ve got all of the above financial stuff ironed out—robust data collection, accurate attribution, detailed segmentation, plenty of historical data, a great understanding of the landscape and future trends, etc. You’re still missing non-financial data points that can significantly boost your CLV’s usefulness:
Customer Engagement Scores measure a customer’s non-purchase value to your business, such as leaving reviews, providing testimonials, and offering product feedback. All of those actions have value to businesses, often helping to convert other customers or improve product offerings, but how do you measure that value to include in your CLV?
Net Promoter Score (NPS) is a widely-used metric to gauge customer loyalty based on their likelihood of recommending a product to someone else. That has obvious value. While NPS itself isn’t that difficult to calculate using surveys, it can be more challenging to convert Net Promoter Scores to dollar values for including in your CLV formula.
Customer satisfaction, though definitely correlated, is separate from both engagement and NPS, which adds another layer of complexity.
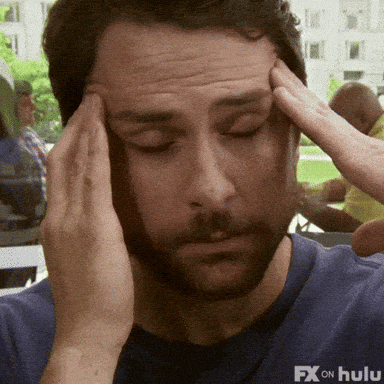
Gif by sunnyfxx on Giphy
Let’s take a quick look at how to calculate those three metrics that comprise CLV using a quick example and then some final thoughts.
Calculating CLV for Tim’s Tiny Top Hats
Tim runs a company that sells tiny top hats for dogs (not for cats, Tim is very insistent on this point). It seems like a dumb business idea until you actually see a dog in a tiny top hat.
Tim’s business is booming, and he’s been the go-to provider of tiny top hats for almost 20 years, so he has plenty of data. He sells top hats in different degrees of tinyness, because dogs come in all shapes and sizes. For this exercise, we’ll look at these two versions:
Top Hat Smol (for the tiniest of dogs)
Top Hat Swol (for the bigger and beefier breeds)
Let’s revisit our CLV formula:
CLV = (Average Order Value x Purchase Frequency) x Customer Lifespan
Due to the nature of the dog top hat business, Tim uses a 2-year window to calculate his Average Order Value (AOV) and Purchase Frequency (PF). Turns out, dogs don’t like wearing top hats and destroy them pretty frequently. Bad for dog owners who need more pics for the Gram. Good for Tim.
Here’s Tim’s sales data for the last two years:
Metric | Top Hat Smol | Top Hat Swol | Smol + Swol |
---|---|---|---|
Period | 2 years | 2 years | 2 years |
Revenue | $36 million | $9 million | $45 million |
Items Sold | 1.8 million | 500,000 | 2.3 million |
Total Orders | 750,000 | 400,000 | 1.15 million |
Note: For simplicity in this example, we’ll assume customers only purchase one type of product—either Top Hat Smol or Top Hat Swol—and don’t place orders with both. If people want, I can use a more complex example for a future email.
Those are some crazy numbers! In the US alone, there are about 90 million dogs. Europe has another 104 million. That’s 194 million pet dogs between just those two regions. 🤯
PSA: 6.3 million animals enter the US shelter system each year with nearly 1 million euthanized. Adopt, don’t shop! My wife and I adopted two shelter dogs a few years back, and I can’t imagine life without them.
Obligatory dog pics: Olive on the left and Archibald on the right.

Olive on the left, Archibald on the right.
What’s that, Bob?

With Tim’s data, it’s easy to calculate a few summary stats, including AOV:
Metric | Top Hat Smol | Top Hat Swol | Smol + Swol |
---|---|---|---|
Period | 2 years | 2 years | 2 years |
Revenue | $36 million | $9 million | $45 million |
Items Sold | 1.8 million | 500,000 | 2.3 million |
Total Orders | 750,000 | 400,000 | 1.15 million |
Items Per Order | 2.67 | 1.25 | 2.17 |
Average Item Value | $20.00 | $18.00 | $19.57 |
Average Order Value (AOV) | $48.00 | $22.50 | $39.13 |
A few comments:
Top Hat Smol significantly outsells Top Hat Swol, both in terms of total orders and items per order. Looks like those tiny, destructive dogs have big dog energy.
Note that there’s a difference between average order value and average item value. Top Hat Smol’s $48.00 AOV is more than double that of Top Hat Swol’s $22.50. Because of the significant volume of Smol, Tim’s top-level AOV is a robust $39.13.
Alright, so we have AOV both segmented by product and overall.
Next up is Purchase Frequency (PF), which is calculated like this:
PF = Total Orders / Number of Unique Customers
“Unique” is the key word there, which means you need to be able to track customers over time. On the surface that seems pretty simple—just aggregate purchases by account to see how often the average account makes a purchase over the designated time period.
However, there’s a wrinkle: What if a customer makes a new account for a subsequent purchase?
In your database, that might look like a new purchase from a new customer when it’s actually a repeat purchase from a single customer. This can be a challenging attribution problem, but it’s made easier with today’s analytics tools.
This specific problem will be the subject of tomorrow’s email, so stay tuned!
For now, let’s assume we have a good idea of the number of unique customers as shown in this condensed table:
Metric | Top Hat Smol | Top Hat Swol | Smol + Swol |
---|---|---|---|
Period | 2 years | 2 years | 2 years |
Total Orders | 750,000 | 400,000 | 1.15 million |
Unique Customers | 500,000 | 350,000 | 850,000 |
Purchase Frequency (PF) | 1.50 | 1.14 | 1.35 |
Once again, for simplicity, we’re assuming customers only place orders for one type of product—either Top Hat Smol or Top Hat Swol—without any overlap.
Now we’ve got PF both segmented by product and overall.
Let’s look at Customer Lifespan, which we’ll shorten as ACL for Average Customer Lifespan (not the ligament).
ACL = Sum of Customer Lifespans / Unique Customers
Sum of Customer Lifespans seems like a pretty weird metric, but it’s basically a way of computing an average.
For example, if I wanted to calculate the average age of my two brothers, I’d do that by calculating the Sum of Brother Ages / Unique Brothers. (lol…”unique brothers”). If my brothers are 34 and 30, which I’m pretty sure they are, the calculation would be 64 / 2 = 32.
So, how do you calculate a customer’s lifespan? Well, it stands to reason you’d need two values: when they became a customer and when they terminated as a customer. Note that this isn’t the only definition, as some companies use time between purchases.
For subscription-based tools, that’s relatively straightforward. For physical products like tiny top hats for dogs, it’s more challenging—especially when the products are purchased less frequently.
(That sounds like the subject of a future email!)
Because today’s email is lengthy, let’s say Tim has performed a fairly robust analysis of his customers using his 20 years of business and determined his ACL is 4 years for Top Hat Smol customers and 3 years for Top Hat Swol customers.
Putting it all together…
Here’s where we stand:
Metric | Top Hat Smol | Top Hat Swol | Smol + Swol |
---|---|---|---|
Period | 2 years | 2 years | 2 years |
Revenue | $36 million | $9 million | $45 million |
Items Sold | 1.8 million | 500,000 | 2.3 million |
Total Orders | 750,000 | 400,000 | 1.15 million |
Items Per Order | 2.67 | 1.25 | 2.17 |
Average Item Value | $20.00 | $18.00 | $19.57 |
Average Order Value (AOV) | $48.00 | $22.50 | $39.13 |
Unique Customers | 500,000 | 350,000 | 850,000 |
Purchase Frequency (PF) | 1.50 | 1.14 | 1.35 |
Customer Lifespan (ACL) | 4 years | 3 years | 3.6 years* |
* Weighted by Unique Customers
Here’s our CLV formula again:
CLV = (Average Order Value x Purchase Frequency) x Customer Lifespan
AOV x PF is the formula for Customer Value (CV) , so let’s start there:
Top Hat Smol: CV = $48.00 × 1.50 = $72.00
Top Hat Swol: CV = $22.50 × 1.14 = $25.65
Smol + Swol: CV = $39.13 × 1.35 = $52.83
Those CVs are over a 2-year period, so let’s divide by 2 to get Annual Customer Values:
Top Hat Smol: $72.00 / 2 = $36.00
Top Hat Swol: $25.65 / 2 = $12.83
Smol + Swol: $52.83 / 2 = $26.41
Now we know the Annual Customer Value, so we can figure out the Customer Lifetime Value by multiplying that by the Average Customer Lifetime:
Top Hat Smol: $36.00 per year × 4 years = $144.00
Top Hat Swol: $12.83 per year x 3 years = $38.49
Smol + Swol: $26.41 per year x 3.6 years = $95.08
See, that wasn’t so hard (he said sarcastically).
A few final thoughts before we wrap up.
What the heck do I do now?
Accurately knowing the value of a customer to your business opens up a world of possibilities:
Compare products, channels, segments, etc.
Better allocate marketing spend
Track CLV changes over time to measure impact of customer retention efforts
Prioritize product development tasks or projects
Measure the impact of different pricing strategies
Forecast future cash flows
Refine customer acquisition strategies
Overall, drive business strategy
There’s a ton more we could cover, but this already has been Data-Driven Marketing’s longest email, so we’ll tackle that stuff in future editions.
Everyone say, “Hi!” to Damon A 👋
Question: Would you rather be able to talk to animals (but they all complain about their day) or be able to speak every language (but only be able to talk about the weather)?
Damon A’s Answer: “Talk to animals because I complain about my day to my pets so they should be able to do the same.“
ChatGPT-Generated Joke of the Day 🤣
Why don't some couples go to the gym?
Because some relationships don't work out.
Suggest a topic for a future edition 🤔
Got an idea for a topic I can cover? Or maybe you’re struggling with a specific marketing-related problem that you’d like me to address?
Just reply to this email and describe the topic.
There's no guarantee I'll use your suggestion, but I read and reply to everyone, so have at it!